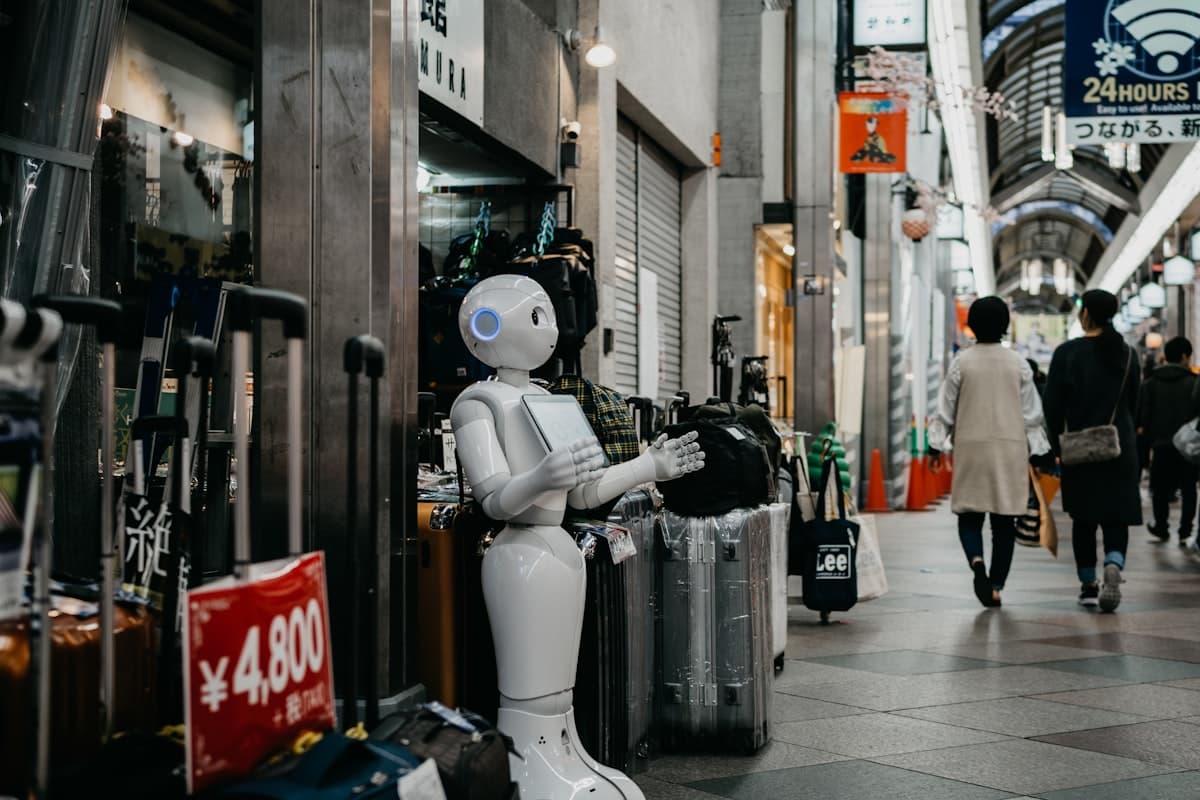
Robot Failure Detection
AI-powered predictive maintenance for industrial robotics
Project Details
Industry
Automotive Manufacturing
Client
Datamole AI
Technologies
Project Overview
At Datamole AI, I implemented advanced anomaly detection algorithms to identify and predict robot failures in automotive manufacturing. This system monitors complex robotic systems in real-time, detecting subtle patterns that indicate potential failures before they occur.
Working closely with industry specialists, our team developed custom AI solutions that analyzed multivariate sensor data from industrial robots to dramatically reduce downtime and maintenance costs.
Technical Approach
The robot failure detection system involved several technical components:
Real-time Data Processing
Pipeline to handle high-frequency multivariate signals from robot sensors in real-time.
Anomaly Detection Models
Advanced algorithms using both supervised and unsupervised approaches to detect deviations from normal operation.
Feature Extraction
Time series feature extraction techniques to identify subtle patterns preceding failures in complex sensor data.
Alert System
Automated alert system with configurable thresholds for different failure types and severity levels.
The system employed a hybrid approach combining statistical methods, deep learning, and domain knowledge to achieve high accuracy in industrial environments with complex noise patterns.
Implementation Challenges
Noisy Data
Working with noisy, high-dimensional sensor data from industrial environments required sophisticated filtering techniques.
False Positives Balance
Balancing false positives (unnecessary maintenance) with false negatives (missed failures) to optimize reliability.
Model Generalization
Developing models that could generalize across different robot types and configurations in varied manufacturing environments.
Interpretable Results
Creating interpretable results that maintenance teams could act upon without requiring data science expertise.
Business Impact
Reduced Downtime
Unplanned downtime in automotive manufacturing lines was reduced by over 35%.
Predictive Maintenance
Identified maintenance needs before catastrophic failures occurred, preventing costly production stoppages.
Cost Reduction
Decreased maintenance costs by enabling targeted, preventive interventions instead of major repairs.
Equipment Lifespan
Extended robot equipment lifespan through early intervention and optimized maintenance schedules.
Production Quality
Improved production throughput and quality by ensuring consistent robot performance.