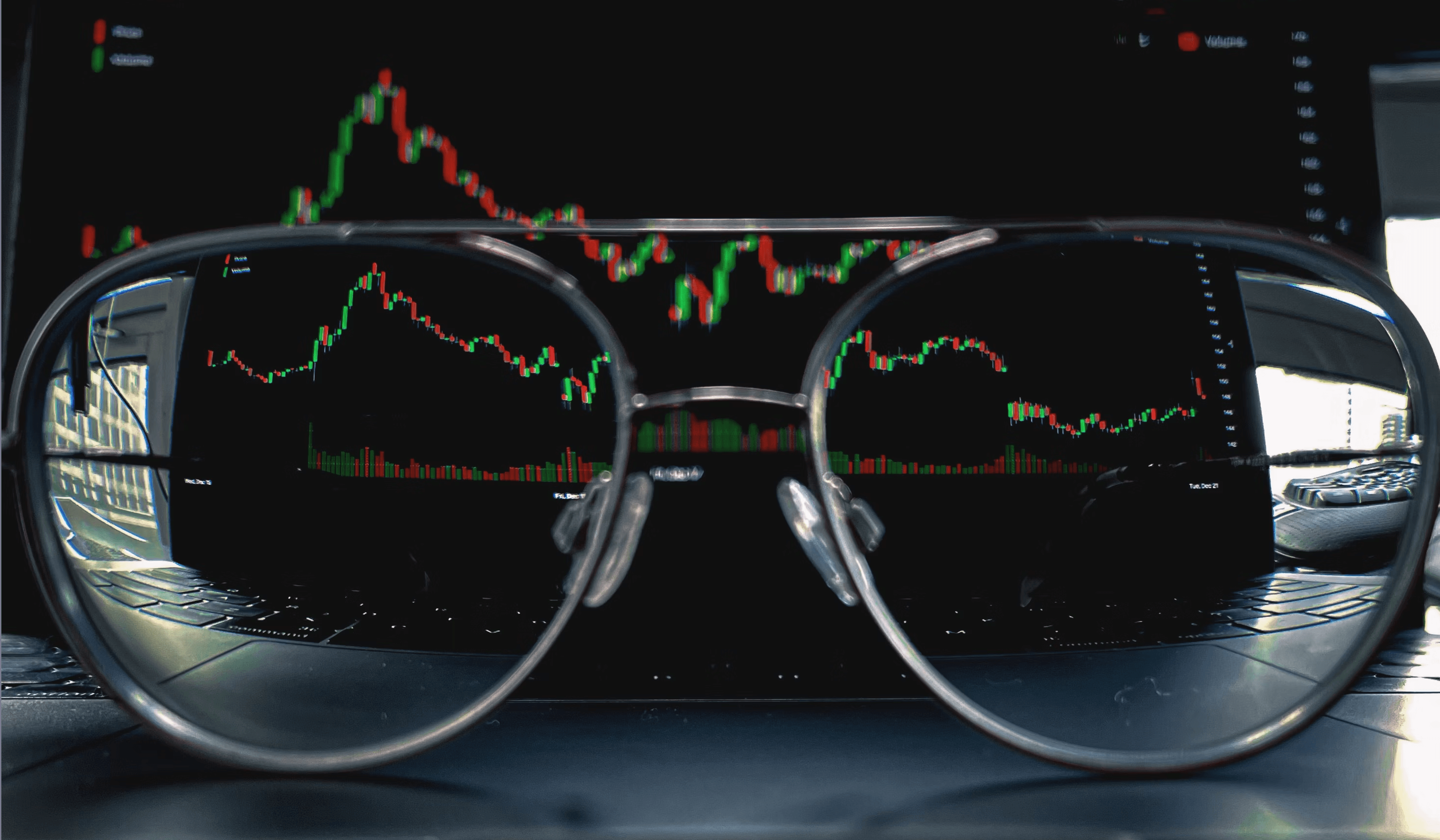
Portfolio Pricing Engine
Risk-optimized pricing framework for energy trading portfolios
Project Details
Industry
Energy
Client
Confidential Utility Partner
Technologies
Project Overview
Developed a modular and portfolio-aware pricing engine for a leading energy provider. This tool integrates risk-adjusted pricing strategies and supports real-time scenario testing for energy tenders.
The Challenge
Traditional industrial-scale energy contract pricing often lacks portfolio context, is reactive, and manually intensive. Key difficulties included:
- •Quantifying financial risk across dynamic, interconnected portfolios.
- •Balancing competitive pricing with adequate risk-adjusted margins.
- •Modeling complex interdependencies between existing and future contracts.
What I Delivered
Simulation Framework Design
Designed and built a simulation framework to test how pricing decisions impact risk across evolving energy portfolios.
Risk Metric Integration
Integrated key financial risk metrics (Value at Risk and Expected Shortfall) directly into the pricing logic to better quantify uncertainty.
Interactive Analysis App
Developed a Streamlit-based application allowing analysts to interactively explore, compare, and visualize different pricing strategies.
Strategic Input
Contributed to the strategic planning for a next-generation pricing engine as part of a broader transformation initiative.
Key Innovations
Portfolio-Aware Modeling
New pricing accurately reflects the current and projected risk exposure of the entire contract portfolio.
Flexible Strategy Evaluation
Enabled dynamic switching between various pricing strategies.
Scenario-Based Testing
Forecasted performance and risk implications across hundreds of simulated market conditions.
Methodological Exploration
To build a robust pricing engine, we rigorously evaluated different modeling techniques, balancing computational speed with analytical depth.
Statistical Approaches
Leveraged historical data analysis and established statistical risk models (like VaR/ES). This provided rapid baseline risk profiling and efficient calculation for standard scenarios.
Monte Carlo Simulations
Employed extensive simulations to model complex portfolio interactions and forecast outcomes under thousands of potential market conditions. This approach excelled at capturing non-linear effects and tail risks.
Key Risk Concepts: VaR & ES (CVaR)
Value at Risk (VaR)
VaR estimates the maximum potential loss for the portfolio over a specific time horizon at a given confidence level (e.g., 95% or 99%). It answers: "What's the most I can expect to lose under normal market conditions?"
While useful, VaR doesn't capture the severity of losses beyond its threshold.
Expected Shortfall (ES / CVaR)
ES, or Conditional Value at Risk (CVaR), measures the average loss expected when the VaR threshold is breached. It answers: "If things go really bad (beyond the VaR level), what's the average loss I can expect?"
ES provides a more comprehensive view of tail risk, crucial for managing extreme events in volatile energy markets.
Integrating both VaR and ES into the pricing engine allowed for a nuanced understanding of potential downside risks, enabling the development of pricing strategies that were both competitive and robust against adverse market movements.
Business Impact & Outcomes
Key Metrics Achieved
Speed Increase
in Pricing Time
Target Reduction
in Portfolio Risk
Growth
in tested scenarios
Strategic Value Delivered
Enhanced Risk Visibility
Provided clearer insights into portfolio risk, supporting strategic hedging and informed tender pricing decisions.
Improved Decision Agility
Enabled faster, data-driven pricing adjustments in response to changing market conditions and portfolio structures.