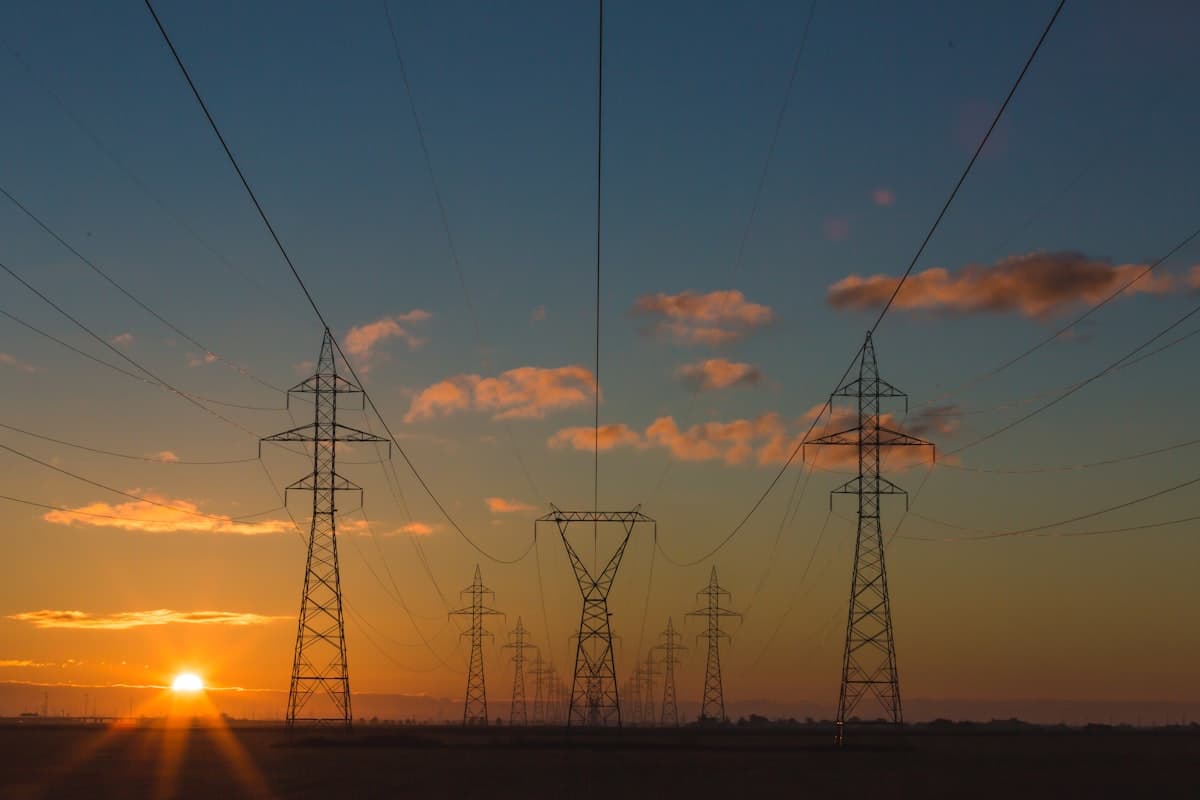
High-Accuracy Forecasting Models
Advanced prediction systems for energy markets
Project Details
Industry
Energy
Client
Energy-tech companies
Technologies
Project Overview
Led the development of advanced long-term load, generation, and price forecasting models for UK energy-tech firms. These systems power core features, support trading decisions, and reduce balancing costs via sub-hourly predictions, achieving a >30% Mean Absolute Percentage Error (MAPE) improvement across hundreds of sites and significantly boosting accuracy and profitability.
The Challenge
Volatility & Seasonality
Managing inherent fluctuations in energy use and renewable generation (solar).
Diverse Data Sources
Integrating complex, high-dimensional data (weather, market, asset specifics).
Scalability
Handling hundreds/thousands of unique sites efficiently.
High Granularity
Maintaining accuracy at sub-hourly levels for operational needs.
Robustness & Efficiency
Developing reliable, computationally efficient, and maintainable models.
Methodological Exploration & Innovations
Specialized Model Components
Advanced Load Forecasting
Techniques sensitive to temporal dependencies and exogenous factors for diverse customer segments.
Weather-Aware PV Generation
Models incorporating weather, panel physics, and site geometry for precise solar predictions.
Battery State Modeling
Predicting degradation and state-of-charge for optimizing storage assets.
Granular Price Forecasting
Sub-hourly market price predictions leveraging market data and volatility modeling.
Modeling Techniques & Innovations
A rigorous evaluation of diverse modeling approaches was key, balancing statistical methods, ensemble techniques, and advanced neural networks. Innovations focused on scalability and learning complex patterns:
Transfer Learning
Applied across sites/tasks in NNs, improving performance and reducing training time, especially for data-sparse sites.
Global Modeling
Developed models learning shared patterns from hundreds of time series simultaneously, enhancing generalization.
MLflow Integration
Used extensively for experiment tracking, model versioning, and results management, ensuring reproducibility.
Hybrid Approaches
Combined strengths of different model classes (e.g., statistical + ML) to capture complex patterns.
Diverse Techniques
Evaluated statistical (ARIMA), ensembles (LGBM), and NNs (RNN, LSTM, Transformer).
Model Architecture
Data Processing Pipeline
Weather Data
Historical Energy Data
Market Signals
ML Prediction Engine
API & Integration Layer
Business Impact & Outcomes
The deployment of these high-accuracy forecasting models delivered significant, measurable value across multiple business areas, headlined by a major leap in predictive performance:
Key Performance Gain
MAPE Improvement vs. Benchmark
Aggregated across hundreds of production sites (Load & Generation)
Enhanced Product Value
Powered core functionality in customer-facing energy management platforms, improving user experience and product stickiness for thousands of users.
Optimized Trading Decisions
Enabled more profitable energy trading strategies through reliable, high-confidence forecasts, directly impacting bottom-line results.
Significant Cost Reduction
Substantially reduced energy balancing costs (estimated in millions annually) through precise sub-hourly predictions, minimizing penalties and optimizing grid interactions.
Improved Market Competitiveness
Provided a distinct competitive advantage for energy suppliers by leveraging superior forecasting technology, achieving over 30% MAPE improvement.